Innovative Feature Engineering - a critical step to connecting Mineral Systems with AI Prospectivity Models.
- Equivest Metals
- Dec 16, 2024
- 4 min read
Updated: Dec 18, 2024
Progress in mineral prospectivity modelling commonly focuses on advancing machine-learning algorithms with limited consideration for improving the representativeness of geological input features to the model. Common inputs are usually oversimplified or biased and often rely purely on direct detection methodology rather than focussing on critical geological processes behind ore formation. To further improve mineral prospectivity modelling, a new approach is needed to draw more value from existing conventional data by applying expert knowledge in creative ways through innovative feature engineering.
What is Feature Engineering in Mineral Prospectivity Modelling?
Feature engineering in mineral prospectivity modelling is the process of transforming data into representations of geological processes that are critical for ore formation. Hence, it is the most powerful when combined with the mineral systems approach, which classifies and quantifies these critical processes and proposes mappable proxies to represent them. Features are then created by geologists and data scientists to populate these mappable proxies. In most prospectivity studies, the majority of created features are direct detection related, such as soil anomalies or chargeability anomalies, and are based on traditional geochemical or geophysical data. This is where clever and innovative feature engineering can be used to leverage existing data to strengthen the mineral system’s representativeness by creating more mappable proxies that express critical ore-formational processes.
Case study: Gravity Worms as mappable proxies
An established and straight-forward example for this are gravity worms. Here, gravity data is processed to extract the maxima of horizontal gradients at various upward continuation levels (see figure 1a). The resulting “worms” are typically associated with large geological discontinuities, such as fault zones and thus represent sites of fluid flow, an essential theoretical criterion for many mineral systems. A good example of the effectiveness of gravity worms is shown in figure 1b, displaying their spatial relationship with orogenic gold systems in the Finnish Central Lapland Greenstone Belt, where all major deposits show close proximity to this engineered feature.
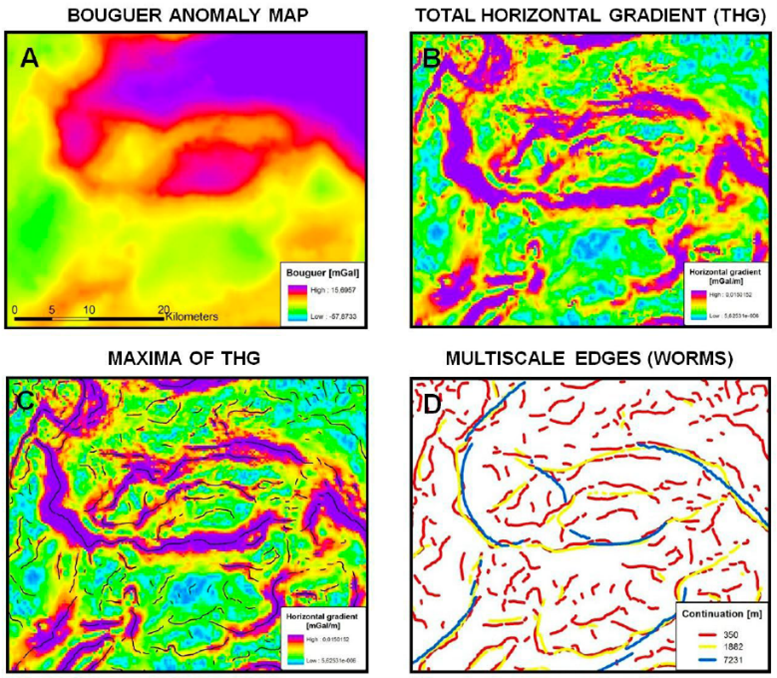
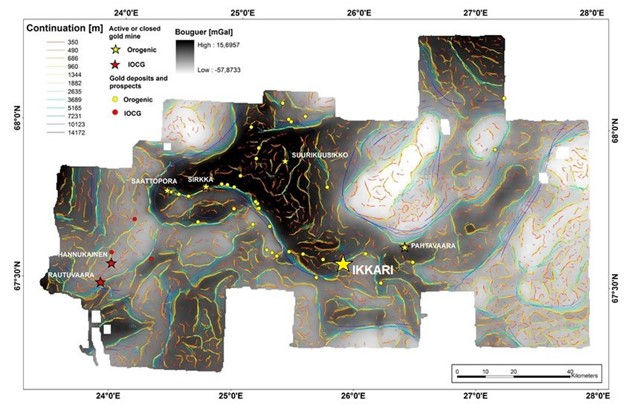
Fig. 1 A) The concept and processing stages of gravity worms. B) Bouguer data, resulting gravity worms, and gold occurrences in the Central Lapland Greenstone Belt, Finland (mod. after Niiranen et al., 2014).
What if the data is lacking?
Not always is feature engineering as straight forward as with gravity worms, especially when one finds themselves in a poorly studied geological system that lacks sufficient high-quality data and where direct mappable proxies are obstructed. At Equivest, we believe that being creative and thinking outside the box is key to make the most of any available data that does exist. As discussed before, to explore for orogenic gold deposits, it is critical to identify large transcrustal structures that facilitate fluid flow. This can prove difficult with poor geophysical data, however, the sought-after lithosphere-scale high strain zones with large vertical displacement are commonly associated with late molasse-type sedimentary basins that are juxtaposed against- and composed of lower rock units. These molasse units can be buffered and turned into a feature to be used as a proxy for structures to determine the rough location and general fertility of the system when no other information is available. A good example for this are the Timiskaming conglomerates in the southern Abitibi Greenstone Belt, Canada which are closely associated with the Larder Lake-Cadillac fault zone, hosting word-class orogenic gold deposits, such as Kirkland Lake (see figure 2).
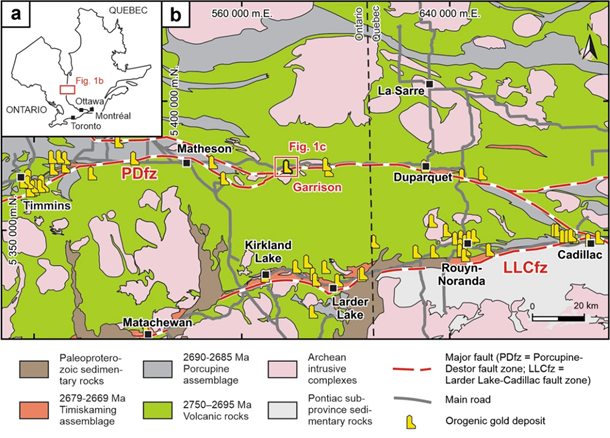
Fig. 2 Geological map of the southern Abitibi Greenstone Belt, Canada. Of note is the spatial association between the Timiskaming molasse unit, the Larder Lake-Cadillac fault (LLCfz), and known orogenic gold deposits (Nassif et al., 2022).
Academic research is slowly moving into improving feature engineering workflows
Due to the focus on machine-learning algorithms in mineral prospectivity modelling, the study of feature engineering has been mostly neglected by public academic research. However, this seems to be slowly changing, with an excellent paper being recently published by Montsion et al., 2024, who introduce several new feature layer ideas and apply them to various mineral systems. As shown in figure 3, the authors find a way to represent geochemical and rheological contrasts of neighbouring lithological units through the clever use of ductile and brittle rock properties (e.g., bulk, shear and compression moduli, and uniaxial compressive strength, frictional, and cohesion coefficients), and semi-discrete element ratios.
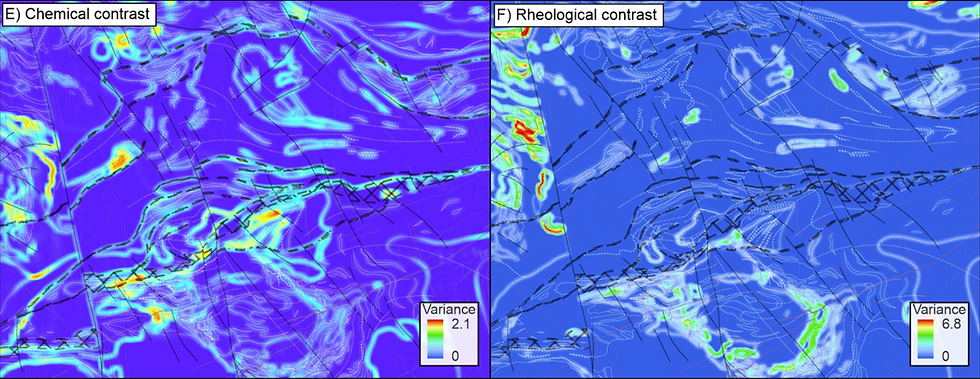
Fig. 3 Feature-engineered chemical and rheological contrasts of neighbouring lithologies (Montsion et al., 2024).
While the shown engineered features still need fine-tuning, they represent excellent mappable proxies for trap-related theoretical criteria that are important for multiple mineral systems, most notably orogenic gold. Also contrasting properties of e.g., porosity, grain density, and magnetic susceptibility can be of importance, when available. Unlike in the oil and gas industry, these type of petrophysical rock properties are often overlooked in mineral prospectivity studies, despite having large potential through geological thinking and smart feature engineering.
To conclude, advancing the capabilities of mineral prospectivity modelling can be achieved in two ways: improving modelling capabilities by advancing ML-algorithms or other methodologies such as common-risk-segment analysis, or by improving the geological relevance of the features that feed the models. While machine-learning algorithms are rightfully becoming ever more widely used and researched, our team believes that innovative feature engineering equally has great potential and remains generally underdeveloped. At Equivest, we are pursuing a combined approach and actively develop both paths in order to pioneer prospectivity studies and to increase the range of what is possible in mineral exploration.
Author: Matthias Mueller, Senior Geologist, Equivest
References:
Comments